
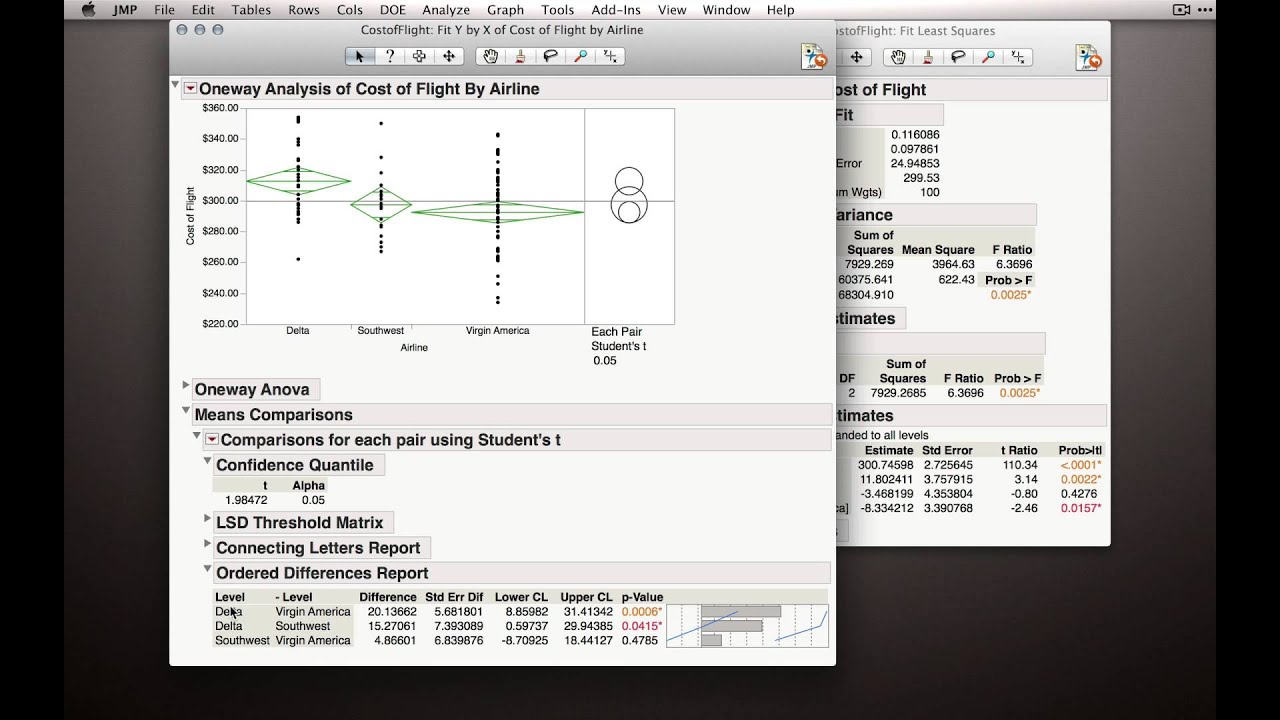
COMPARE R AND JMP SOFTWARE
This can be troublesome in an ANOVA framework, because you will typically have to adjust the output in the standard ANOVA table when you run the model without the intercept in order to get valid interpretations (because the logic of standard ANOVA tables as output by most statistical software is typically assuming you have included the intercept).JMP is an on-premise data analytics solution that helps scientists, engineers and data explorers understand complex data relationships and visualize them via interactive dashboards. With the intercept, parameters are interpreted as differences with respect to a reference category, whereas without the intercept parameters are interpreted with respect to the conditional expectations of the levels of the categorical variable. ANOVA is a special case of linear regression where the only predictors are categorical in this case, the model with and without the intercept are actually equivalent, the only differences being in how you interpret the parameters. In a linear regression framework, for example, the intercept helps ensure that the Gauss-Markov theorem is satisfied by forcing the residuals to be mean 0, which further ensures that your parameter estimates are unbiased. Simply, it never HURTS to have the intercept in the model. Typically, I would advise that you do not remove the intercept unless you really know what you are doing. Basically I'm asking whether the Sum/Contrast portion of the analysis can make more or less sense to look at depending on whether a "baseline" is present or not? But also, how would I go about investigating the change over time of my between-subject factors when I don't have a baseline? Is the contrasting from RM ANOVA still the correct way to go, and if so how would I follow up on it in case of interaction between a between-subject factor and the within-subject factor? I briefly considered a regular 4way ANOVA as a solution, but since "time" is a within-subject factor, this would not be right either. Still, I would like to investigate whether there are changes with my between-subject factors over time, so some sort of accounting for time is necessary. On the other hand, the sum-portion of the analysis seems to make alot more sense here because it is similar to looking at the total energy intake for the entire period - which is also interesting.
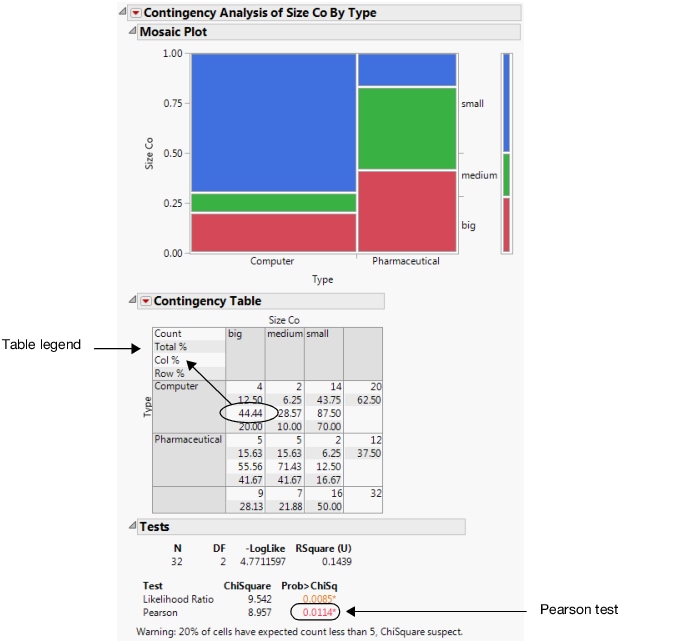
In that case I'm not sure the individual contrasts make as much sense, since the measurements from week 1 don't constitute a baseline for comparison, they are just another datapoint with the same status as the others. But let's say I'm investigating energy intake and have measurements for the first 4 weeks. Because of this, analyzing the change/contrast makes alot of sense. In the example above, the contrasting makes perfect sense because the "untreated" measurement serves as a baseline for the measurement of the "Acutely treated". Another thing I'm running into with RM ANOVA is situations where I'm not sure the contrasting makes as much sense as otherwise.
